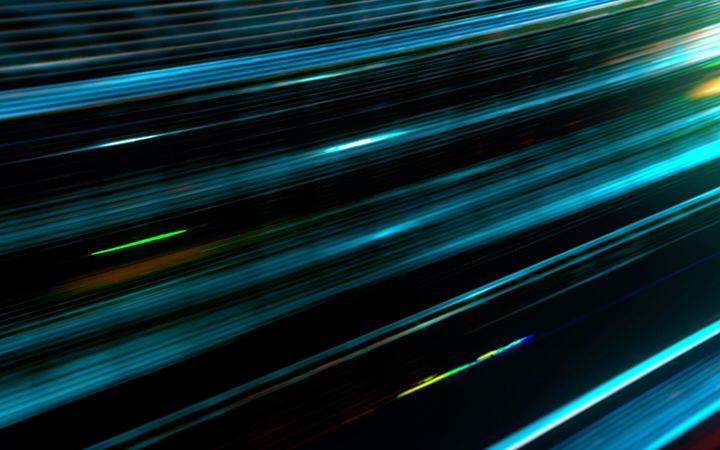
Streamlining literature reviews with AI and subject-matter expertise
Literature reviews underlying management, policy, or regulatory decisions are often complex and cumbersome processes. The ever-increasing volume of specialized literature and the speed at which it must be reviewed—accurately—can be a challenge. And the need to integrate different types of literature from different disciplines exacerbates this challenge and underscores the need to have an expert hand guiding the process.
Thankfully, AI capabilities can help make the process more streamlined and efficient. For 20 years we have combined technology with subject-matter expertise to prepare literature reviews for federal clients, and we have innovated with machine learning and natural language processing tools for over a decade. Now, adding to that experience with generative AI (Gen AI), we tailor solutions to the use case at hand—because literature reviews are anything but cookie cutter.
Here are three benefits to combining subject-matter expertise with a range of AI tools to optimize literature reviews.
1: Tailored solutions
A partner who understands your discipline and the problem you are trying to solve will be able to help you compare different tools and select the one that best meets your needs. For example, a more traditional AI classifier that uses natural language processing and machine learning models may be the best fit for scientific literature because the language in those abstracts tends to be highly standardized and templated—a good use case for older AI models. In contrast, economic papers are far less structured and predictable and can benefit from the sophisticated semantic understanding and deep learning that Gen AI brings to the table.
By knowing how the reports are set up, a subject-matter expert can help you determine the right tool to use—and then train it appropriately to get accurate results.
2: Accurate outputs
How do you know that your literature review will stand up to public scrutiny and stringent outside review? Our subject-matter experts partner with AI and data science practitioners behind the scenes to conduct rigorous assessments to ensure accuracy. We start with fully human-curated datasets and look at accuracy and precision metrics to understand performance on a given problem. Then we make sure the model settings have optimized the accuracy and precision of that set.
As a result, we may know that our model is about 80% to 90% accurate, which helps us understand the risks involved in our approach and how to mitigate them. We always put human-in-the-loop safeguards in place to make sure we’re catching errors and ensuring the accuracy of the literature review outputs.
3: Greater transparency
Transparency is a federal requirement for literature reviews and central to decision-making. As we navigate classification challenges, the traditional performance metrics we’ve come to expect from machine learning models may no longer suffice. Instead, we need to illuminate our methodology, offering a clear window into the process.
AI, and the solution overall, should provide transparency with explainable steps and results, reflecting the outputs back and showing how the data was used at each step. This means articulating each step taken, demystifying AI’s decision-making, and confirming that the journey from data input to result is clear for both literature review teams and third parties. It’s about fostering trust and replicability through clarity, reflecting thoughtful and transparent application of Gen AI at every output.
We bake transparency into our literature review process, prioritizing AI explainability and ensuring our clients can trust the results and make informed decisions with confidence. Our machine learning tools document everything from the literature search strategy to the systematic review steps, providing a holistic solution to manage, report on, and visualize your assessment.
“We have a lot of AI tools in our toolbox, and we know when to apply the correct ones—and how to do it safely. We understand the challenges that come from less structured documents and grey literature and the importance of modifying your approach to managing and processing them in a systematic way.”

A powerful tool in your toolbox
Litstream is a systematic, collaborative literature review solution that supports the process from end to end with transparent process tracking, customized approach prioritization, and automated flows from one step in the review process to the next.
It builds on experience and knowledge from subject-matter experts across key disciplines but can still be customized by review managers so that the review steps are fit-for-purpose and literature is prioritized. That way, mission and team leaders can be sure that team members have what they need to succeed and are truly spending their time most effectively.
Literature reviews can be daunting, particularly given the speed at which new data is created and disseminated. But by utilizing the right tool, for the right stage of the process, for the right piece of literature, guided by a partner with real working knowledge of your unique needs, reviews can be easier and faster than ever.